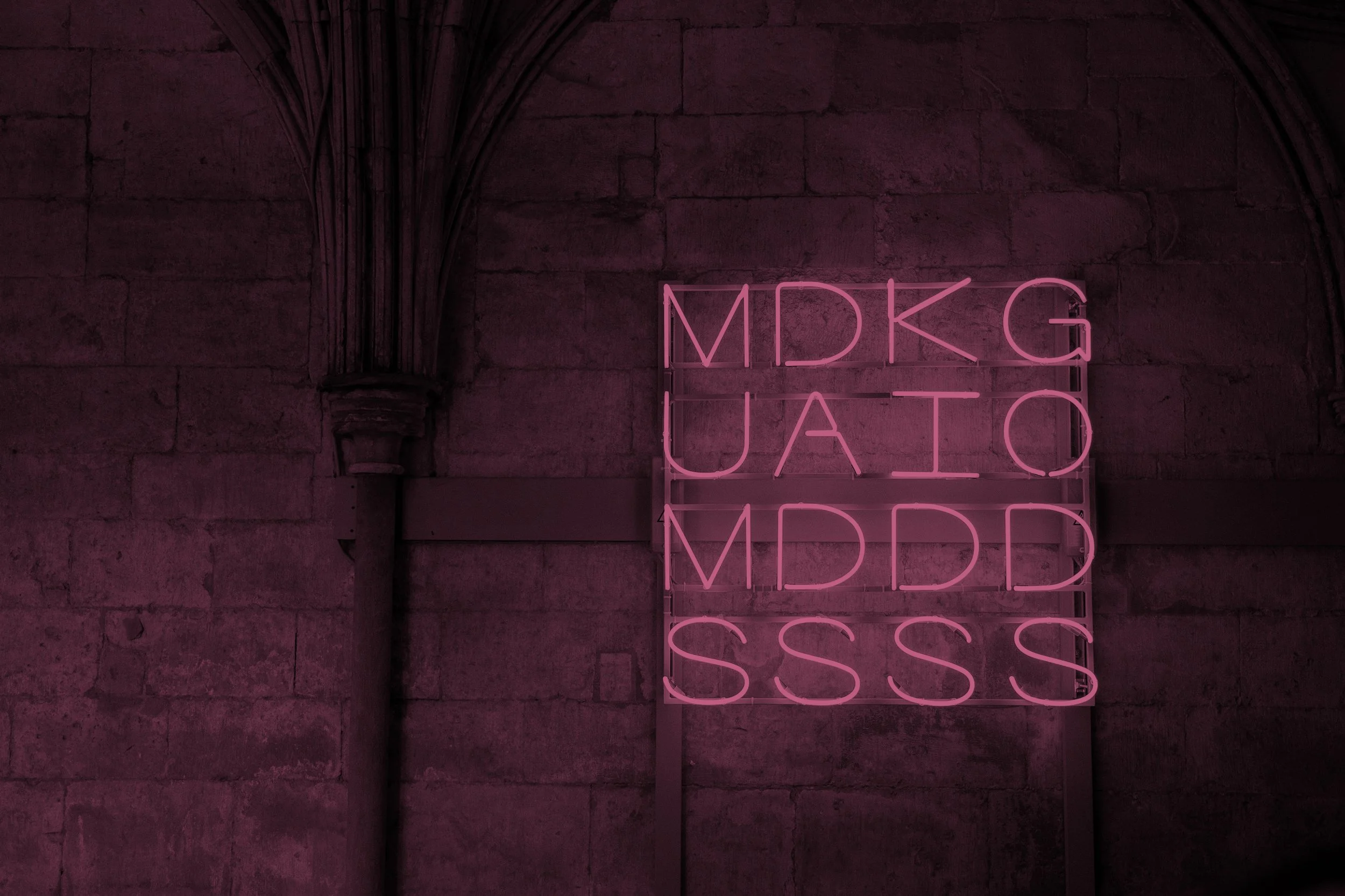
Abstraction in LANGUAGE
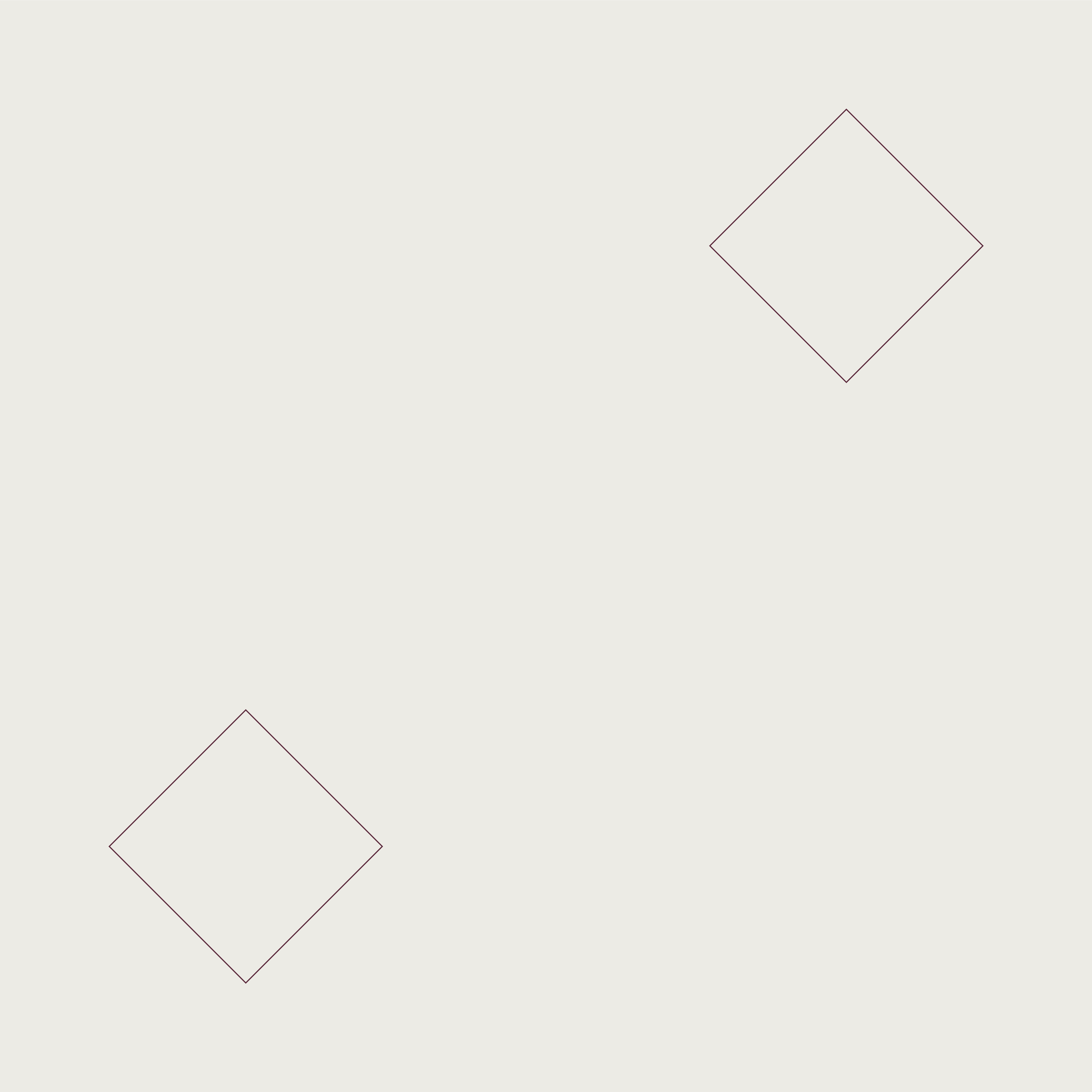
Abstraction in LANGUAGE
“Good writing skills”, broadly speaking, include guidelines like “be clear”, “use examples”, “avoid vague language”. In a widely cited essay, George Orwell (1946) provides several examples of bad texts, and then argues that two qualities are common to all of them. The first is staleness of imagery; the other is lack of precision.
Staleness of imagery may be interpreted as lack of clarity and vividness, a flaw that can be contrasted by using concrete words that evoke vivid images. Lack of precision may be interpreted as vagueness or genericity, a flaw that can be contrasted by using more specific and precise words.
The goal of this part of the project ABSTRACTION is to understand how word concreteness and word specificity influence the perceived clarity and informativeness of different types of texts, written for different readers. Consider the following example, in which the SARS-CoV-2 virus is explained to three different types of reader.
Children: SARS-CoV-2 is a very little bug that can get into people’s nose and mouth, and make them sick.
Adults, non-experts: SARS-CoV-2 is a virus that can cause severe and potentially lethal respiratory problems.
Adults, specialists: SARS-CoV-2 is a positive-sense single-stranded RNA virus that enters human cells by binding to the angiotensin converting enzyme 2.
The first definition uses words that are quite generic but concrete. The second uses abstract words, but still quite generic ones. The third uses abstract and concrete, highly specific words. Using words at the right level of concreteness and specificity is arguably crucial to ensure that a message reaches effectively the intended reader. Texts that do not fulfill the readers’ expectations of clarity and informativeness produce misunderstandings, frustration, boredom, or delays, depending on the context.
ABSTRACTION will investigate how concreteness and specificity function and interact in different types of text, written for different types of reader through a series of automated linguistic analyses based on machine learning, surveys, and statistical analyses.
The results of these studies will be published in academic journals that can be found on the academic website of this project, and will be summarized here on this website in short articles.